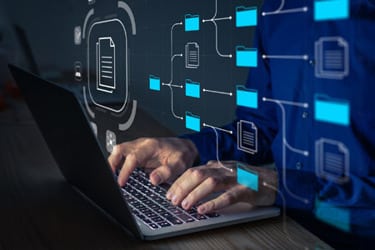
By Eyal Wultz, President & Co-Founder, and Bremer Louw, Vice President, Biometrics Operations & Business Development, Bioforum
Data capture, analysis, and reporting are critical activities during the clinical trial process, as this information provides clinical researchers with a reliable and high-quality source for validating a product’s successes and failures. Yet, without a standardized method for data collection and, eventually, submission to the regulators, the integrity of the data – and the success of the trial – can be at considerable risk. Recognizing this, the FDA mandated in 2014 that all clinical trials beginning after December 17, 2016, must submit data in a standard supported by the Clinical Data Interchange Standards Consortium (CDISC).1
CDISC’s standard for tabulated clinical data is the Study Data Tabulation Model (SDTM), which provides a standard structure for organizing and formatting data to streamline processes in collection, management, analysis, and reporting.2 However, the increasing complexity of clinical trials and regulatory requirements are posing significant challenges to producing SDTM deliverables within limited timelines and budgets. So, too, is the task of finding and retaining qualified and experienced programming resources. Therefore, how can sponsors ensure SDTM compliance while still maintaining the efficiency and speed necessary to meet their trial timelines and milestones?
Standardizing Clinical Data In A Complex Landscape
While innovation across today’s pharmaceutical industry has led to new and exciting possibilities in patient care, it is also increasing the complexity of clinical trials due to a wide range of factors. These factors include the wider use of adaptive trial designs, wearable devices, real-world data, basket trials, a growing number of data sources, and an evolving landscape of guidance documents and therapeutic area user guides. As a result, clinical trials are producing higher volumes of data than ever before. A recent study by the Tufts Center for the Study of Drug Development found that Phase III clinical trials currently generate an average of 3.6 million data points, which is three times the data collected by late-stage trials only 10 years ago.3 Thus, with so many different therapeutic areas and study designs, clinical trial submissions can look vastly different from one to the next, which will affect the quality, cost, and timeline of a sponsor’s application.
When the FDA mandated the requirements for standardized study data in 2014, its goal was to achieve better data quality, transparency, and interoperability between clinical research and regulatory submissions.1 Specifically, it indicated that the standards “provide a consistent general framework for organizing study data, including templates for datasets, standard names for variables, identifying appropriate controlled terminology and standard ways of doing calculations with common variables.”4
The agency said data standards “also help FDA receive, process, review, and archive submissions more efficiently and effectively.”4 Japan’s Pharmaceutical and Medical Devices Agency later followed suit by requiring data in SDTM format for new drug applications after April 1, 2020.5 And while there are many benefits to standardizing study data, implementing SDTM also comes with its own challenges that sponsors must be aware of to ensure they are planning appropriately ahead of time.
The Bottlenecks Of SDTM Today
The top priorities for SDTM implementors are guaranteeing data correctness and completeness while remaining compliant with changing regulatory guidelines. However, there are many project management elements they must take into consideration while doing so. Time is, of course, the most essential, as part of a drug’s success in the market depends on how rapidly a sponsor can transition from idea to commercialized product. SDTM is an important milestone on this critical path because it provides the sponsor with the data they need to understand whether they are meeting the primary and secondary endpoints of their study. The earlier a sponsor can set up SDTM during a clinical study, the more possibilities there are to use it for standard visualizations of the data, such as data review and cleaning as well as gaining valuable insights into the study itself. Finding and retaining qualified statistical programmers for SDTM is also a challenge, as it requires them to be specialized in not only the SAS programming language but also the data standards, clinical data, and therapeutic area expertise.
In the end, the speed at which data analysis and interpretation is completed is what determines whether a sponsor is ready to apply for FDA approval and, just as importantly, how quickly they are able to do it. SDTM activities are also tedious, as the process of converting the captured data from multiple sources (i.e., electronic data capture [EDC] data, laboratory vendor data, patient diaries, and more) and mapping it into a standard format is a manual task that requires a significant amount of time and number of resources, which contributes to the overall cost of the program. Utilizing a system that could streamline these steps through automation would increase efficiency and, thus, speed to market.
Streamlining SDTM Activities
Historically, the pharmaceutical industry has lagged in automation adoption when compared to other industries. Yet, over the last decade, there has been an increased application of automated solutions throughout the drug development and manufacturing life cycle. This is due to the competitive edge it offers its adopters by minimizing the risk of human error, ensuring constant control throughout production, and achieving better productivity. Clinical trials are no exception. Integrating automation, especially in data collection, management, analysis, and reporting, can yield significant advantages that allow sponsors to accelerate timelines, lower costs, and improve quality.
Today’s regulatory requirements call on quality control processes that can ensure high levels of data quality and reliable statistical results, all of which add to the clinical program timelines. Traditionally, this is achieved through a practice called double programming, where two independent programmers will create the same deliverable and then compare results to determine if they are the same. If so, the assumption is that everything is correct. This is an inefficient approach that can add 25% more time to the SDTM conversion process and does not always ensure a high level of quality. In many cases, especially with junior resources, the focus of double programming is incorrectly seen as establishing a successful comparison between two programmers rather than making sure the data is complete and compliant to the data standards. Not doing so, though, can threaten compliance and leave potential gaps the sponsor may not realize exist until it is too late. Another example of a gap in the traditional way of delivering SDTM data is that the current systems do not provide an automated audit trail, leaving version control of programs and data for the programmers to complete manually, which results in a lack of traceability and accountability and, possibly, deficiencies during an audit.
Innovative Solutions To Improve Speed And Quality Of Data
Leveraging machine learning and a purpose-built graphical user interface instead could eliminate double programming and, alternatively, offer a rigorous review process that provides detailed visualizations of the conversion flow and logic. This would assure all expected data was transferred to SDTM as well as accurate compliance to the data standards. In addition, validations that are typically executed after programming and often cause rework can instead be integrated into the SDTM conversion process and performed in real time to automatically detect and address changes from the previous run.
Machine learning could also automatically provide suggestions for different mappings for various scenarios that are based on information gained from past studies. Finally, with the click of a button, an automated system for SDTM could provide the valuable documentation that must be included in the SDTM submission package to the FDA, which includes an annotated Case Report Form (CRF), a define-XML, and the clinical study data reviewer’s guide. The above streamlining enables a process that can occur in a repeated and expected fashion, eliminating much of the human intervention and reducing the conversion timeframe by half.
Overall, the responsibilities associated with SDTM can be overwhelming and so, too, can the challenges associated with its implementation. Sponsors with limited resources may consider outsourcing clinical trial activities to a CRO for tasks such as SDTM, but one must ensure the CRO they use relies on efficient methods that offer the edge they need in an increasingly competitive market. By combining the advantages of the automated SDTM system described above with the benefits of outsourcing to a qualified and experienced CRO, sponsors can accelerate their drug discovery and development timeline, ultimately improving quality and costs as well as their success rate. In cases where sponsors
are currently performing their own SDTM conversion programming, outsourcing this work instead allows SDTM to function as a commodity, giving sponsors the ability to utilize their programmers for other tasks, such as downstream analysis.
Automation With The Future In Mind
With the pharmaceutical landscape evolving at a rapid pace, overcoming SDTM bottlenecks is essential to achieving the efficiency and accuracy needed when managing clinical trial data. That is why Bioforum has designed an automated system for producing SDTM deliverables within reduced timelines and budgets. With all the features described above, Bioforum’s automated system is purpose-built for converting clinical data into the CDISC SDTM format. It combines next-generation technology with SDTM and data experts, allowing us to address customer needs today while providing scalability for tomorrow’s growth.
For more information, contact Bioforum experts now at https://bioforumgroup.com/contact-us/.
References
1. FDA. (February 2015). Electronic Submissions – The Requirement for Standardized Study Data. https://www.fda.gov/media/91152/download
2. CDISC. SDTM. https://www.cdisc.org/standards/foundational/sdtm
3. GlobeNewswire. (January 12, 2021). Rapid Growth in Clinical Trial Data Volume, According to Tufts Center for the Study of Drug Development. https://www.globenewswire.com/news-release/2021/01/12/2157143/0/en/Rising-Protocol-Design-Complexity-Is-Driving-Rapid-Growth-in-Clinical-Trial-Data-Volume-According-to-Tufts-Center-for-the-Study-of-Drug-Development.html
4. FDA. (September 19, 2022). Study Data Standards Resources. https://www.fda.gov/industry/fda-data-standards-advisory-board/study-data-standards-resources
5. PMDA. New Drug Review with Electronic Data. https://www.pmda.go.jp/english/review-services/reviews/0002.html